“Cleanlab is well-designed, scalable and theoretically grounded: it accurately finds data errors, even on well-known and established datasets. After using it for a successful pilot project at Google, Cleanlab is now one of my go-to libraries for dataset cleanup.”
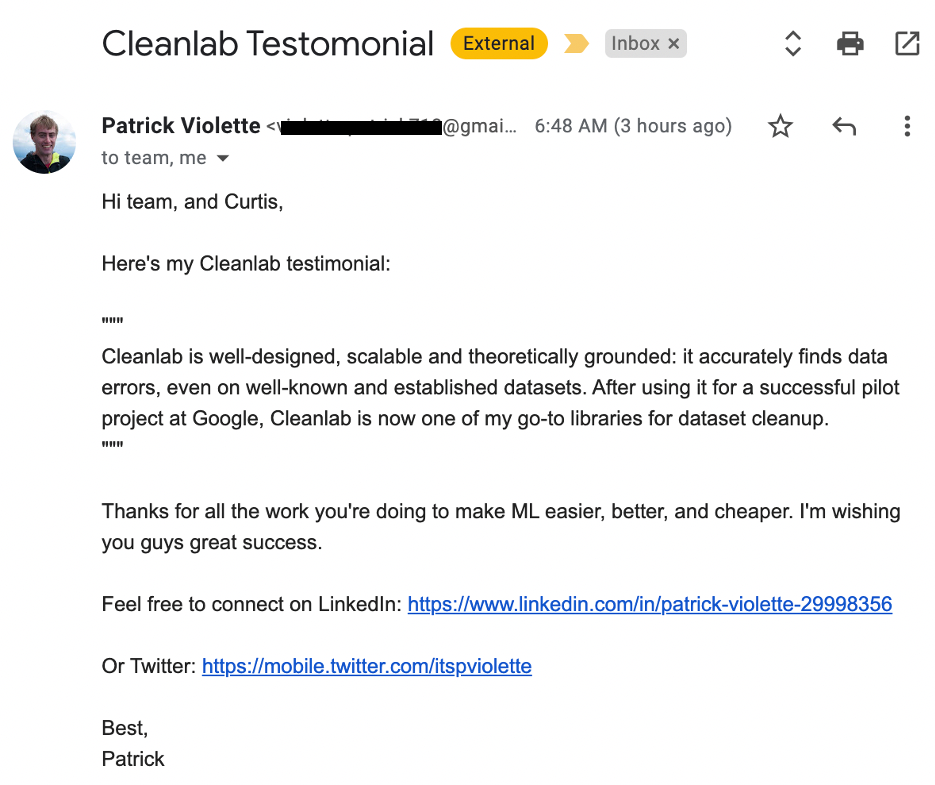
“[Cleanlab] really opened my eyes to the whole idea of confident learning. I’m currently checking out the Cleanlab package and I really appreciate the clean api. A lot of what we do involves questionable labels so we’re looking into making Cleanlab a standard processing step whenever we get labels.”
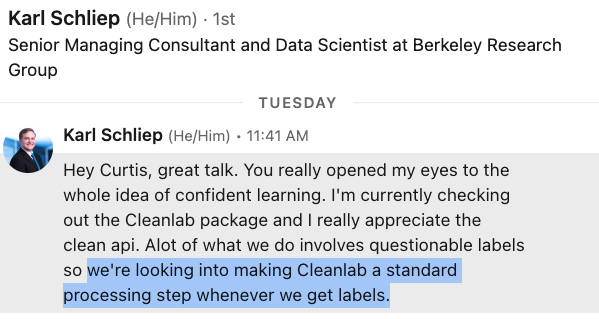
“CleanLab helped us reduce the uncertainty of noise in the tags. This process enabled us to train the model, update the training set, and optimize its performance. The goal was to reduce the number of labeled transactions and make the model more efficient, requiring less time and dedication. This allows data scientists to focus on tasks that generate greater value for customers and organizations.”
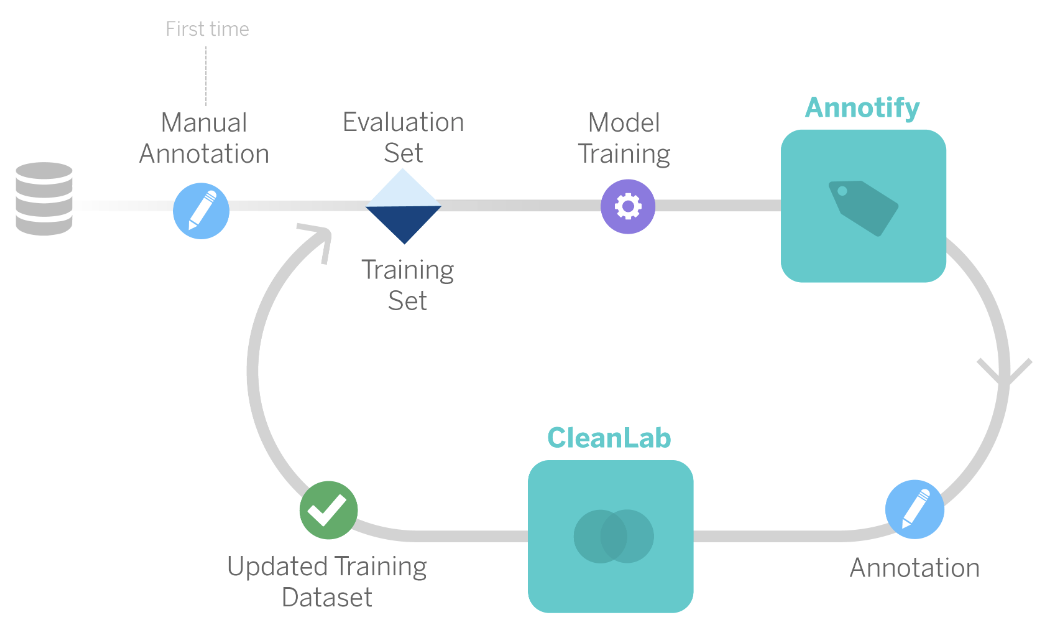
“[Cleanlab] allows me to upload a dataset and obtain a ranked list of all the potential label issues in the data in just a few clicks. The label issues can then be assessed and fixed right away in the GUI… Cleanlab Studio is a very effective solution to calm my nerves when it comes to label noise.”
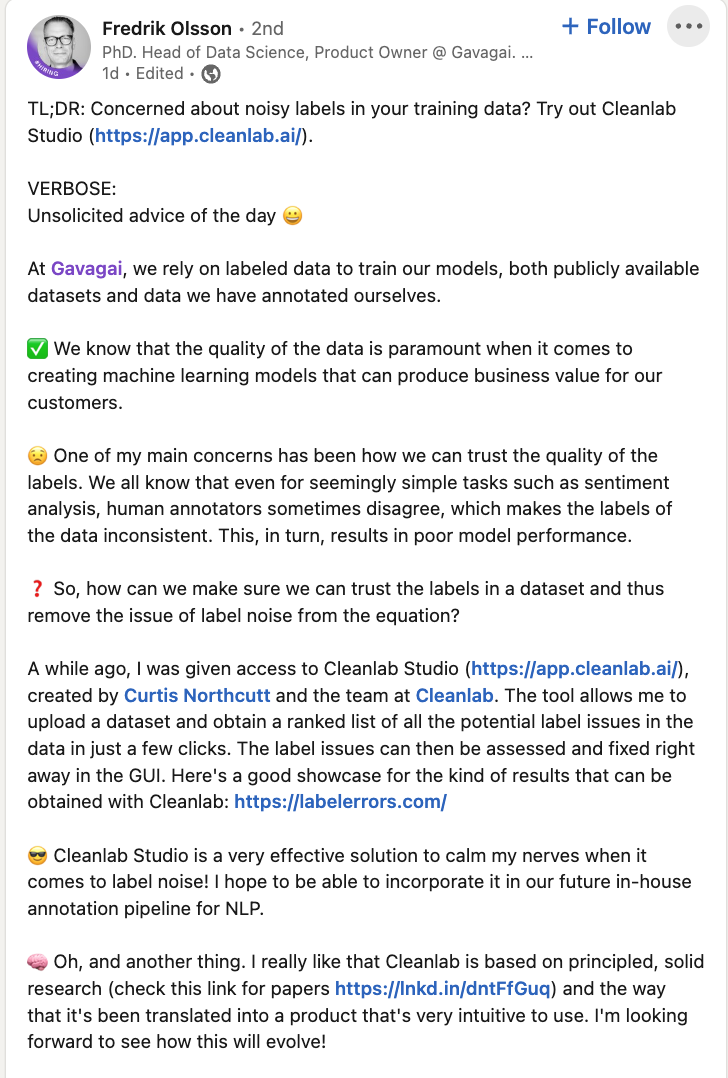
“We have used Cleanlab to clean SRL benchmark dataset. The result was impressive. […] There was a significant improvment in the F1 score when training with the corrected data. 0.5 or 5% marginal improvements in both dev and test folds.”
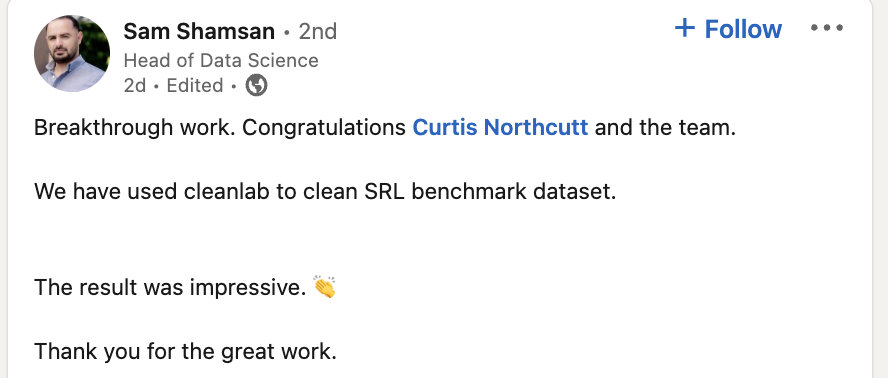
“Recently took part in a new kind of ML competition based on Andrew Ng’s idea of shifting focus from model-centric to data-centric AI. Found cleanlab, a useful package in supporting this data-centric movement. It is based on the field of confident learning and helps to detect and learn in the presence of noisy real world labels. Some of the most common datasets like ImageNet, CIFAR and MIST have errors too.”
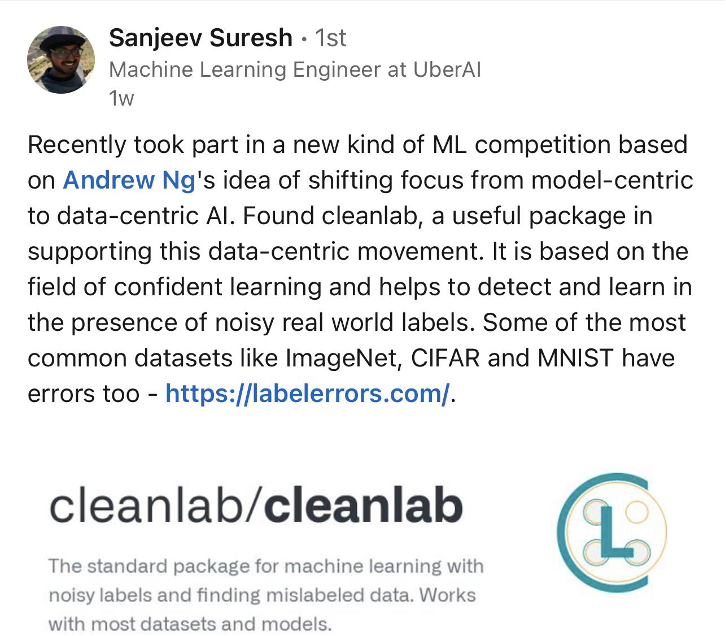
“Curtis Northcutt and Anish Athalye at MIT and Jonas Mueller at Amazon trained a model to identify erroneous labels in popular datasets such as ImageNet, Amazon Reviews, and IMDB. Accuracy on a test set that’s rife with errors is not a true measure of a model’s ability, and bad labels in the test set have a disproportionate impact on bigger models.
It’s time for our community to shift from model-centric to data-centric Al development. Many state-of-the-art models work well enough that tinkering with their architecture yields little gain in many problems, and the most direct path to improved performance is to systematically improve the data your algorithm learns from.”
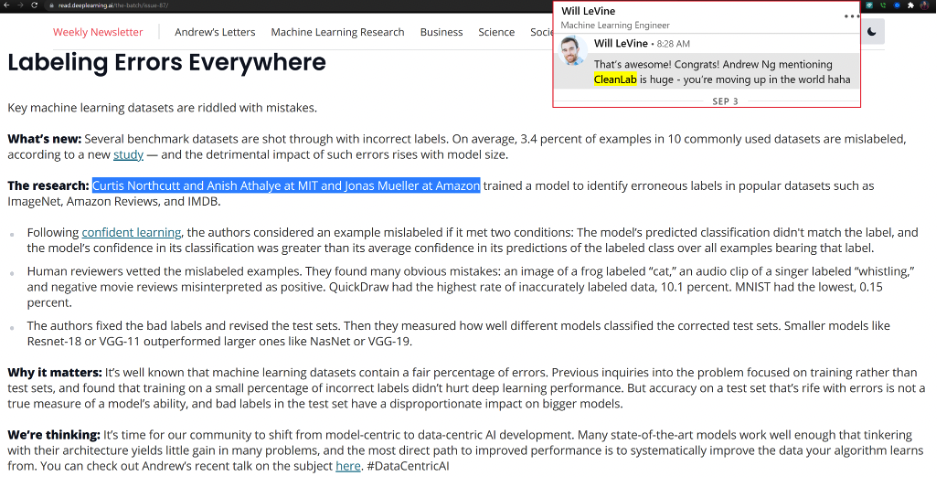

“Noise in the labels … hearing this probably triggers cold chills to any scientist willing to train a model for production purposes. Today, I’ve discovered an amazing library that implements Confident Learning: Cleanlab! It identifies label errors in datasets, based on the principles of pruning noisy data, counting with probabilistic thresholds to estimate noise, and ranking examples to train with confidence.
Cleanlab makes it so simple to use, it’s well maintained, and with production standards. This thing works like a charm and can benefit your training in the context of high noise in your labels! One of the rare work/libraries that I wonder why it took me so long to discover…”
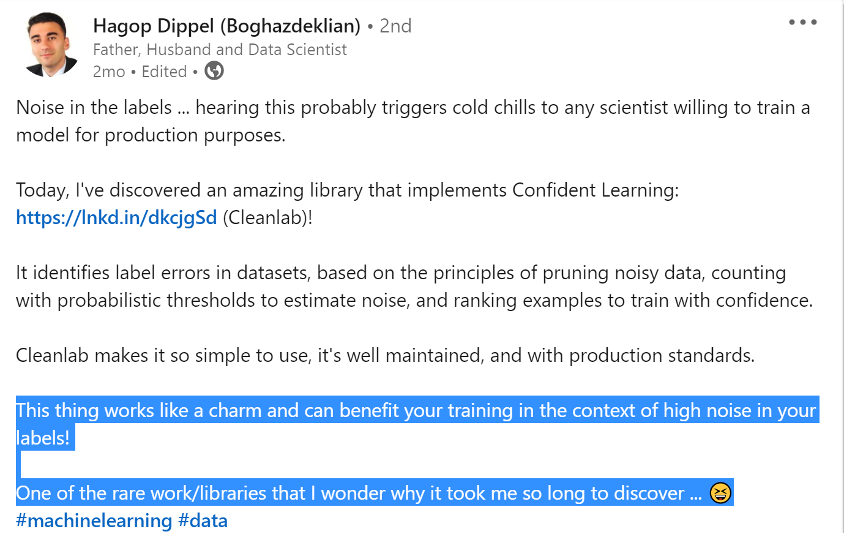
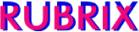
“As shown recently by Curtis G. Northcutt et al. label errors are pervasive even in the most-cited test sets used to benchmark the progress of the field of machine learning. They introduce a new principled framework to “identify label errors, characterize label noise, and learn with noisy labels” called confident learning. It is open-sourced as the cleanlab Python package that supports finding, quantifying, and learning with label errors in data sets Rubrix provides built-in support for cleanlab and makes it a breeze to find potential label errors in your dataset.”
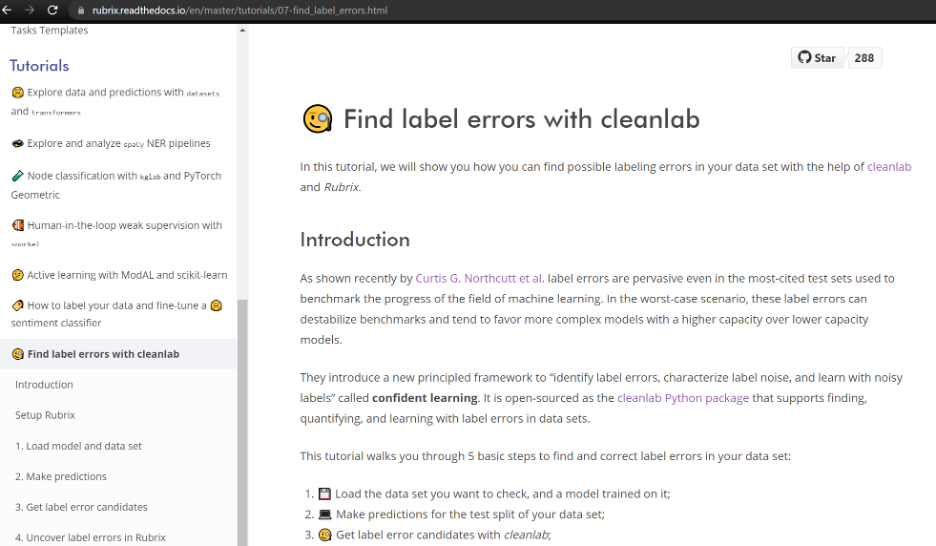
“I think CleanLab is a very interesting idea and is one of the few methods I’ve seen that is both theoretically justified and work in practice.”
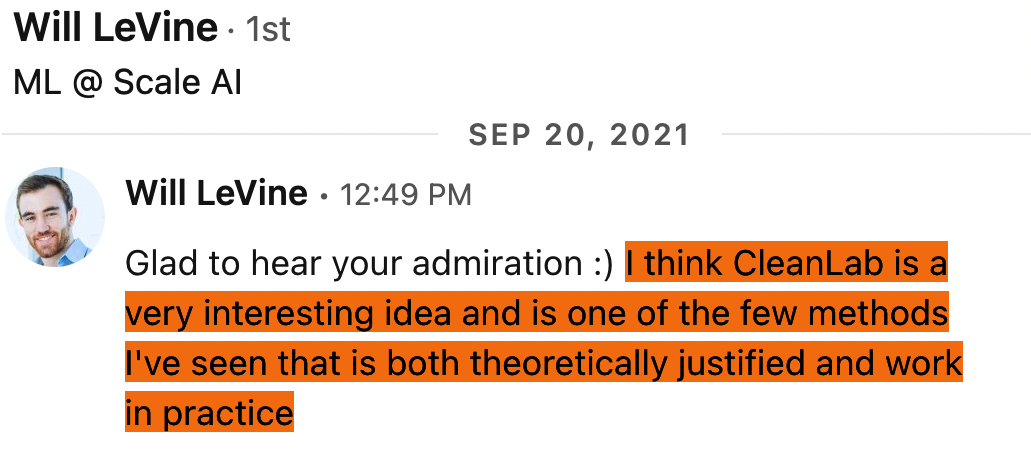
“A couple weeks ago, I found out about a Python library called “cleanlab” that can help identify mislabeled training data. […] Tonight, I took the sentence-labeled training data and threw it at cleanlab to see how well confident learning could identify the incorrect labels. These results look amazing to me. […] I like this. I really need to dig into this. If nothing else, this can help identify training data to TOSS if you don’t want to automate correction.”
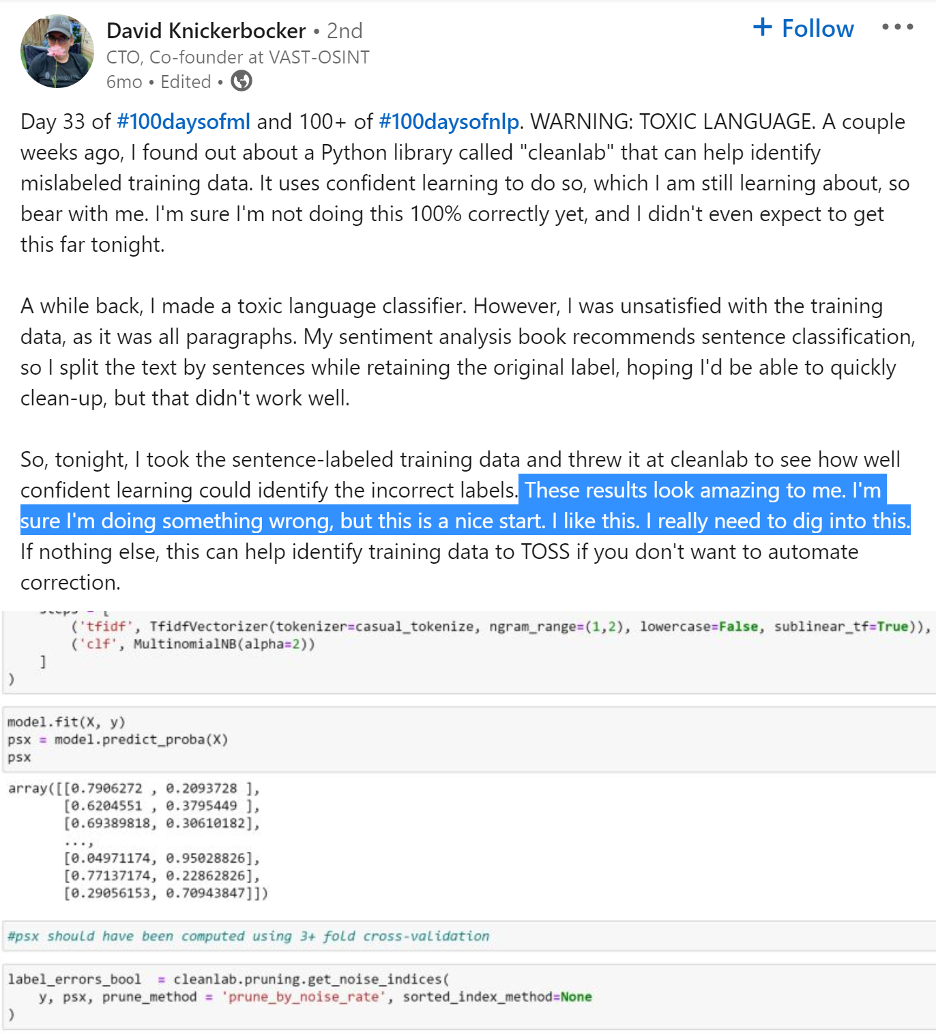
“Improving your training data is more important than using the latest “state-of-the-art” mode. Here’s a very simple trick: use cleanlab, a Python package for machine learning with noisy labels and finding mislabeled data.”
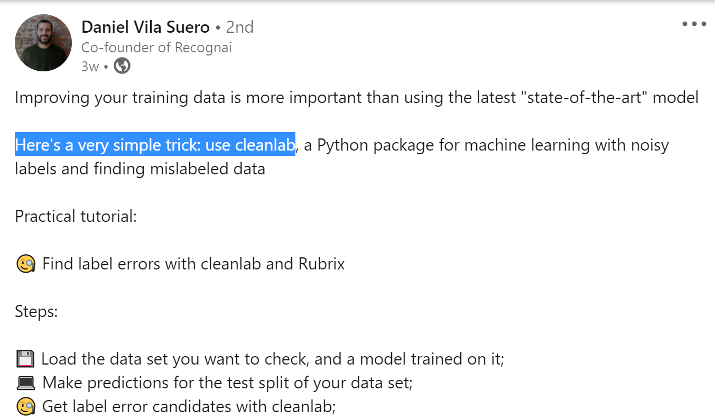
“More people should do check their labels more frequently. Anybody is free to try out any trick that they like, but if you’re looking for a simple place to start, check out the cleanlab project. It’s made by the same authors of the labelerrors-paper and is meant to help you find bad labels. I’ve used it a bunch of times and I can confirm that it’s able to return relevant examples to double-check. […] The disclaimer on the Google Emotions paper checks a lot of boxes, but imagine that in the future they’d add ‘we checked out labels with cleanlab before releasing it’. For a dataset that’s meant to become a public benchmark, it’d sure be a step worth adding.”
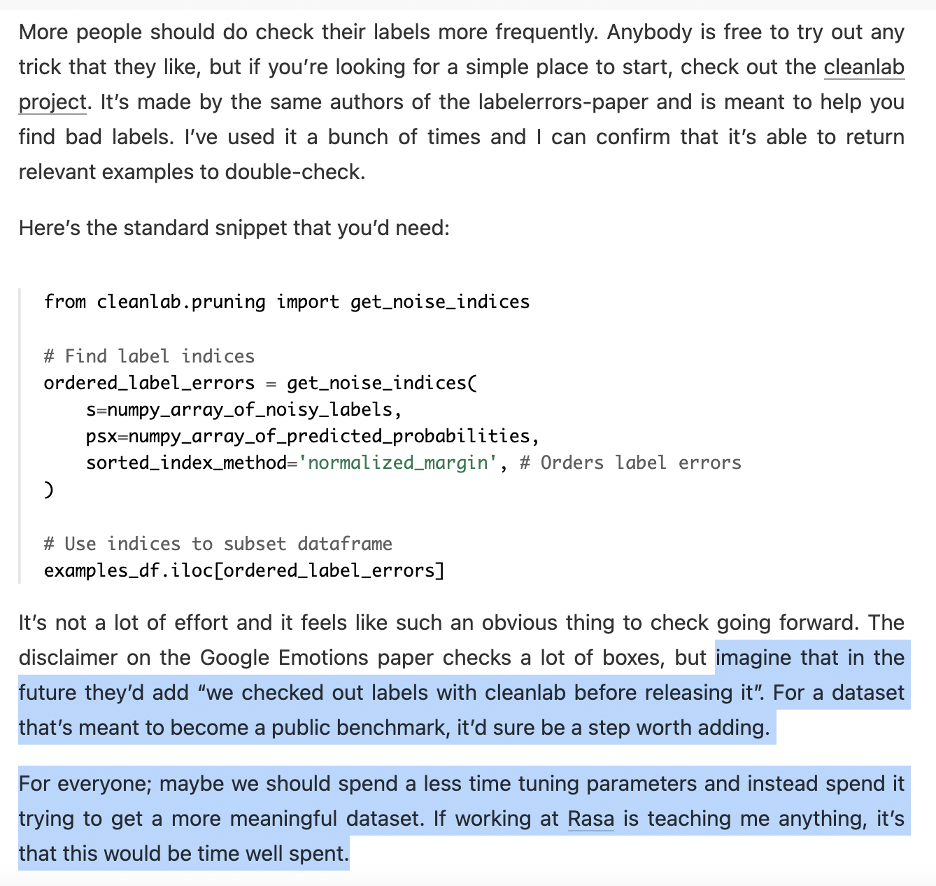
“One of the challeges in AML is that labels are not clean and prone to human error. […] There has been extensive reasearch into learning with Noisy labels. One of the most usable approaches has been described in this paper and implemented in [the cleanlab] open source python package. […] First and foremost cleanlab can be used to identify the noisy labels.”
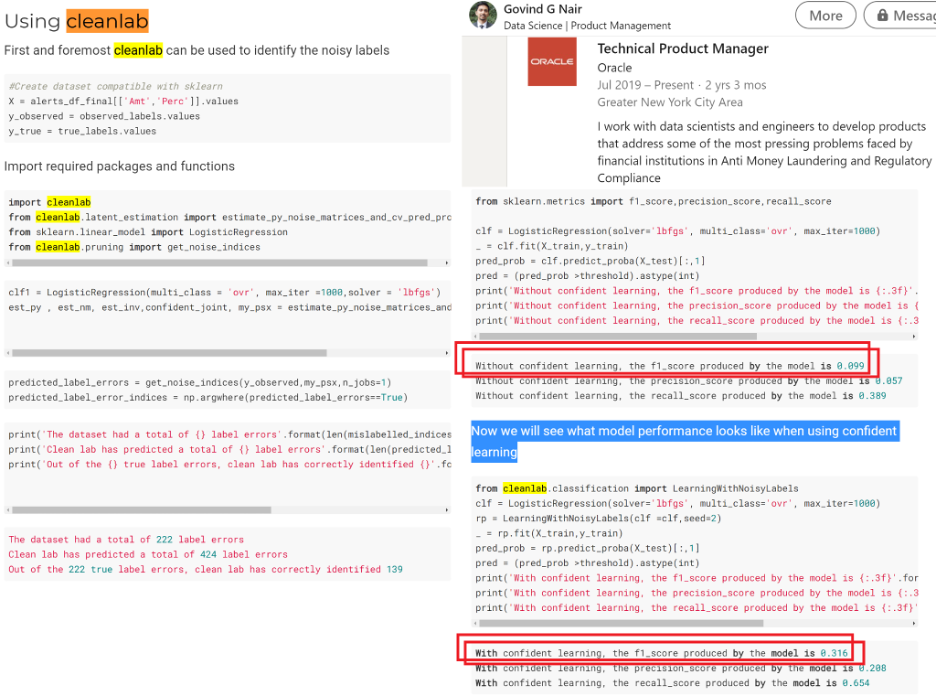
“This feels like something profound in supervised AI! Perhaps those last few percentage points of many benchmarks are only a cleanlab away from SOTA results. Awesome work!”
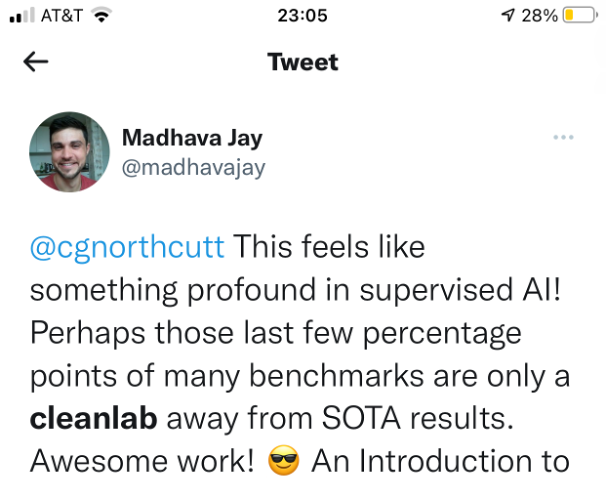
“Most of the datasets are noisy and incorrectly labelled. Sharing an example notebook to show case how to detect and clean noisy labels in the disaster tweets text dataset can improve the accuracy(0.79 -> 0.85). This is done after detecting and removing the noisy labels using cleanlab.”
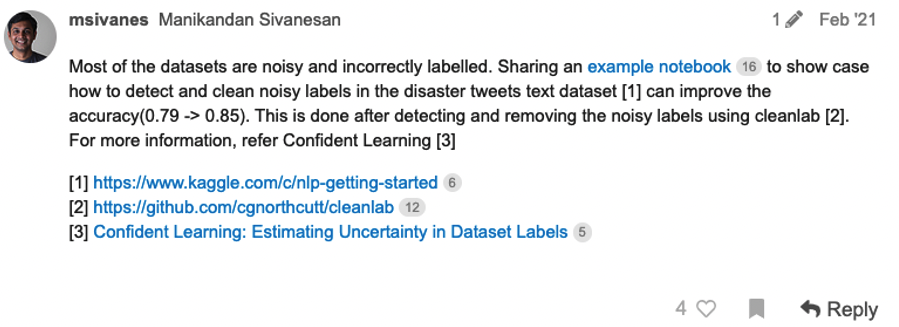
“Thanks for sharing about the cleanlab package @Addison! I prototyped it late last week on the outputs of one of our classification models, and its outputs were of course not perfect, but definitely useful. It can help us identify: potentially duplicate classes, potentially mislabeled examples, and noisy classes that should potentially have their examples moved out into different already existing classes e.g. legacy superset classes. One thing I really like about that package is how model-agnostic it is. All that’s needed is a sequence of labels.”

“Use the Cleanlab library to compute outlier scores based on model output (embeddings, probabilities) and inspect outlier candidates. Use the Cleanvision library to extrapact typical image issues (brightness, blurr, aspect ratio, SNR and duplicates) and identify critical segments through manual inspection.”
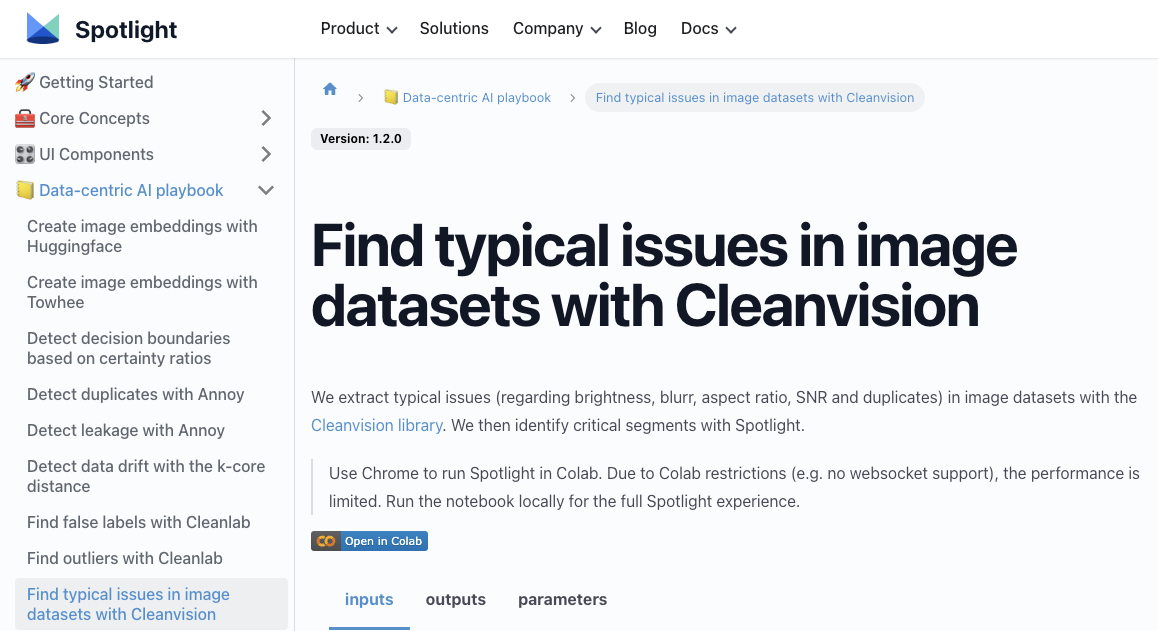
“Knuckle bump to [Curtis Northcutt] Anish Atalye, and Jonas Mueller for the paper diving into this. https://arxiv.org/pdf/2103.14749.”
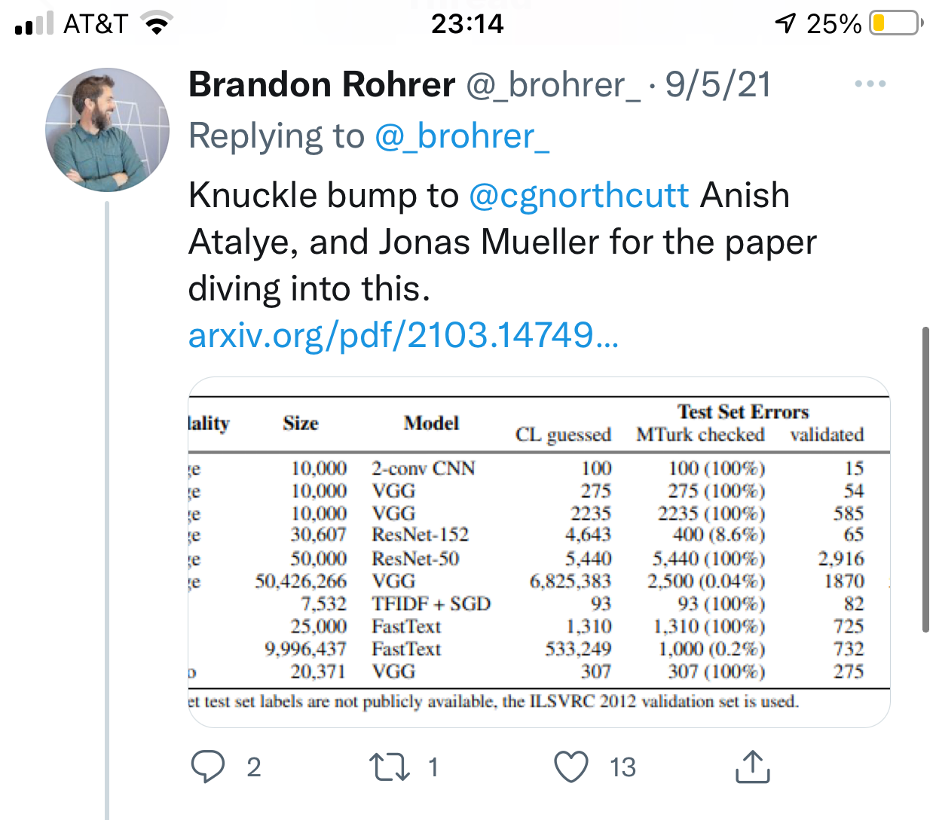
“Our data is particularly “dirty” involving many mislabeled data points and in my research of how to alleviate this issue I came across the Clean Lab ai. I’m very interested in your work and how it may apply to our project.”
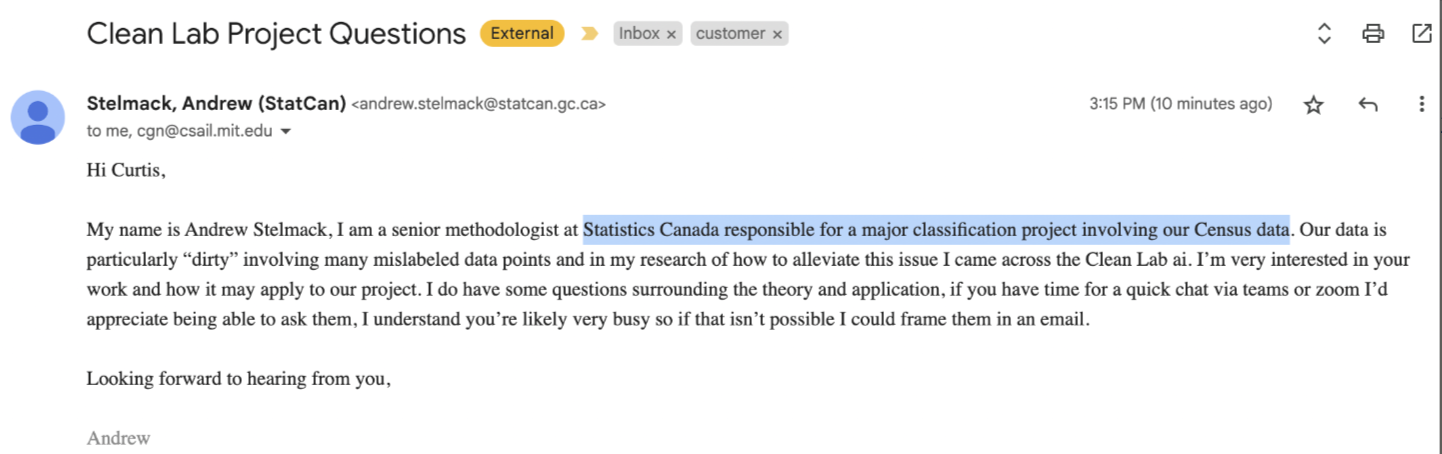
“Cleanlab is immensely helpful for my work. Thank you for that. Love the story behind the company and your work! Keep going with this great tool!”
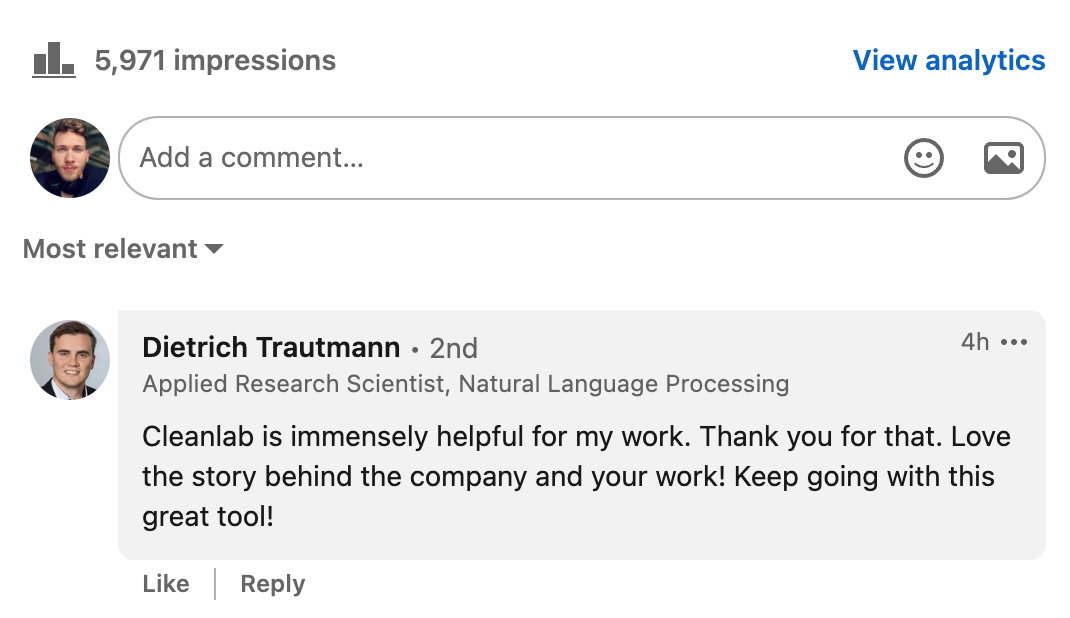
“We propose a novel mean-teacher-assisted confident learning framework to robustly exploit the noisy labeled data for the challenging hepatic vessel segmentation task. Specifically, with the adapted confident learning assisted by a third party, i.e., the weight-averaged teacher model, the noisy labels in the additional low-quality dataset can be transformed from ‘encumbrance’ to ‘treasure’ via progressive pixel-wise soft-correction, thus providing productive guidance.”
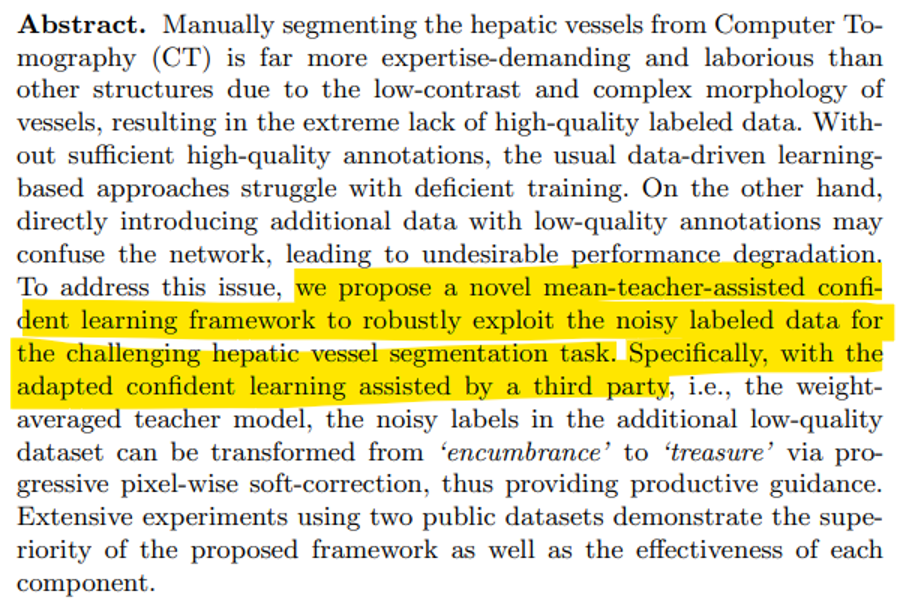
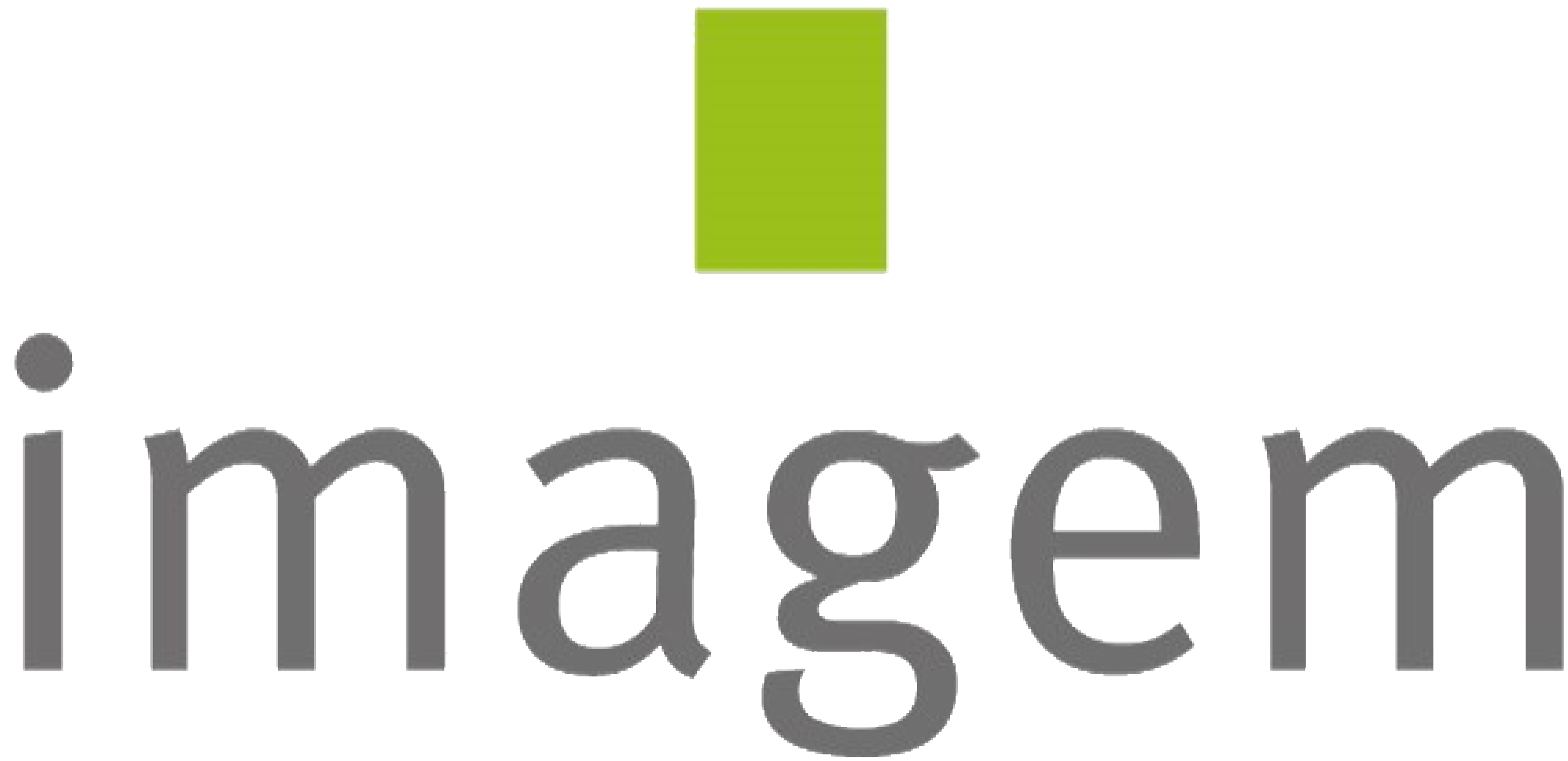
“CleanVision helped me improve the quality of my image data and, as a result, the accuracy of my model. This tool has proven to be invaluable to me, it is helping me to improve the data quality of computer vision projects, allowing us to effectively address a variety of common issues in our imagery dataset.”
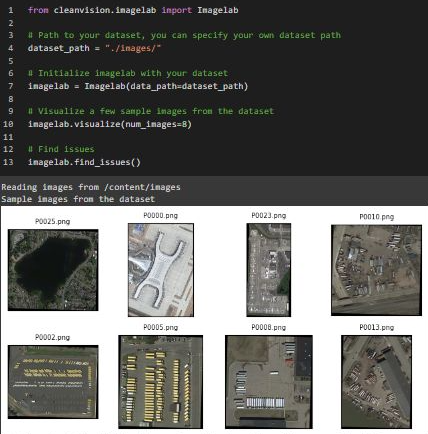
“We used Cleanlab to quickly validate one of our classifier models’ predictions for a dataset. This is typically a very time-consuming task since we would have to check thousands of examples by hand. However, since Cleanlab helped us identify the data points that were most likely to have label errors, we only had to inspect an eighth of our dataset to see that our model was problematic. We later realized that this was due to a post-processing error in the dataset — something that would otherwise have taken a much longer time to notice.”
“I used an open-sourced library, cleanlab, to remove low-quality labels on an image dataset. The [ResNet] model trained on the dataset without low-quality data gained 4 percentage points of accuracy compared to the baseline model (trained on all data).”
“Our approach is based on the Cleanlab implementation of active learning for data annotation. Our datasets include over 18 million depth image frames and 22 million patient face image frames extracted from videos. It is not practical to annotate the entirety of these massive datasets. Active learning is an important machine learning technique that involves an iterative process to choose most informative data samples to be labeled. Another important aspect is the annotator quality, which can significantly impact the training effectiveness of the machine learning model.”
“I demonstrate the use of cleanlab, a confident learning implementation, to easily find noise in the data. Confident learning provides a solid foundation for analyzing a dataset of noisy or OOD samples — a technique that’s quite effective for multi-class approaches, with the evolving support for multi-label classification.”
“My Cleanlab Studio experience was very positive. Very surprised how fast and easy it was to get results. Most work was transforming metadata into CSV file. You have really great product here, formatting the data for upload is really the only work needed to analyze/improve any data. You can take somebody who has no Computer Science background and they can have big impact where they previously could not play with and improve data directly. Customer support experience was also great, all of my questions/issues were quickly resolved by Cleanlab engineering team.”
“CleanLab was used to remove approximately 5,000 scenes that were considered noise. I did some experiments, including some that weren’t included in the final submission. The model trained on the cleaned dataset increased LB in the stand-alone model, but not much when ensembling. The following two points can be made from this evaluation: CleanLab is effective (+0.003), and large effect of ensembling FixedLen and VariableLen (+0.01).”
“Cleaning Data Labels — A Problem for Today and Tomorrow […] Since we don’t have an a priori gold label set to evaluate our confidence scores, we’ll use Cleanlab’s processing of the predicted probabilities from cross validation segments to arrive at a reasonable approximation for a set of gold labels. Thus, we can reduce the downstream burden of human evaluation by finding and relabeling the worst performers automatically. […] Our pipeline and Cleanlab’s algorithm detected between 1,354 and 1,993 label issues (depending on the classifier used) which were then relabeled, or moved to an unknown category for further inspection.”
“If the classifier is trained with these noisy images directly, its performance could be degraded. In view of this, we attempted to find label errors in the image dataset with an open source tool cleanlab”, a framework powered by the theory of confident learning. Specifically, we trained multiple ResNet50 image classifiers to compute the predicted product category probabilities for all the training samples in a |cross-validation manner. Then the cleanlab tool could utilize the matrix of predicted probabilities to find noisy samples, ordered by likelihood of being an error. We removed the top 10% noisy samples from the training set.”
“Recently last year MIT researchers created Cleanlab as a tool to find label errors in image, text, and audio datasets. The scholars tested their tool with the 10 most popular image, text, and audio datasets, including Cifar-10, ImageNet, IMDB, and AudioSet. In one of their publications, they show how these datasets, which are widely used to benchmark new and improved Machine Learning algorithms, have errors. […] These new labeling error detection algorithms are recent, and their effectiveness has to be tested in environments external to academia.”
“The simplest approach is to stop training a model early, before it’s memorised the training set, and then use this model to run inference back over its own training set. The frames with the largest disagreement between the original labels and the model’s predictions are likely to include incorrect annotations. Send the top X of these to your labelling tool, and correct where appropriate. See cleanlab to get started.[…] 90% of the gains can be achieved through a pretty simple set-up built around open-source tools like cleanlab, CVAT, Voxel Fiftyone and maybe the odd Streamlit app.”
“This is a weakly supervised multi-label classification problem and a code competition. Given images of cells from our microscopes and labels of protein location assigned together for all cells in the image, Kagglers will develop models capable of segmenting and classifying each individual cell with precise labels. If successful, you’ll contribute to the revolution of single-cell biology! We use the Kaggle provided dataset and the public dataset to train and evaluate using different model architectures. The public tools used include Fastai, Opencv, CellSegmentator, Cleanlab, etc.”
“Wikidata is a great resource of free data. However to interact with it meaningfully most people will find it necessary to clean the data. For more details on how some data was labeled manually, how BERT embeddings were used to build a classifier and how Cleanlab was used to detect problematic labels, please visit the ML-You-Can-Use notebooks regarding the label provenance.”
“Similar to the deep learning framework PyTorch, Cleanlab is a framework for machine learning and deep learning with error labels. […] It can be used to describe, find and learn label errors. The cleanlab Python package is free and open source.”
“It’d be a shame if our machine learning models are merely optimal because they overfit on the bad labels. That’s why we’re going to explore heuristics to find bad labels in our training data so that we may try to improve the quality of our training data. This will also give us the opportunity to explore cleanlab, which is made by the creators of the label errors website to help spot bad labels.”
“At TikTok, I deploy models for video tagging at an enormous scale. My expertise lies in Large-scale ML Ops operations. I’ve witnessed the transformative impact of enhancing data quality, often overshadowed by flashier methods. At TikTok, I actively utilize Cleanlab to swiftly identify incorrect annotations, consistently delivering high-quality models on schedule.”
“We use the Python package cleanlab which leverages confident learning to find label errors in datasets and for learning with noisy labels. Its called cleanlab because it CLEANs LABels. cleanlab is: fast (single-shot, non-iterative, parallelized algorithms), robust (provable generalization and risk minimization guarantees, including imperfect probability estimation), general (works with any probabilistic classifier), and unique (the only package for multi-class learning with noisy labels or finding label errors for any dataset / classifier).”
“As the course comes to a close, I would like to take a moment to express my sincerest gratitude for your guidance and support throughout the lectures. It took me about a month to complete all nine lectures, including labs and notes, but I can say that this path has been the most illuminating experience in my educational life. Your unwavering dedication to teaching and commitment to my learning experience has not gone unnoticed. You have inspired me to continue learning and growing beyond the classroom. Your generosity with your time and knowledge has made a significant impact on my journey. Thank you once again for all that you have done for us.”
“It takes time and effort to check each image and manually remove the noise image, but it seems to be easy by using cleanlab. I think it is also convenient that it can be used regardless of the framework.”
“I’m just starting to get the hang of this and read on how it works. But right now from the first results it looks like pure black magic… So thank you for this!”
“I collected custom image data from the internet for one of my pet projects. When i went through the data, i saw a lot of duplicate images. Initially i was deleting them all manually (not fun at all). This library was a game changer. Just one function and everything is done.”