HOW CLEANLAB CAN HELP
Find tens of thousands of data errors.
Large-scale datasets used in enterprise data analytics and machine learning are often full of errors, leading to lower reliability, lost productivity, and increased costs. With Cleanlab, you can automatically find and fix issues in data at scale, effortlessly curating high-quality datasets.
ImageNet is the most famous computer vision (image recognition) dataset with millions of images. Cleanlab Studio automatically found thousands of data errors in their dataset, like label issues, outliers, ambiguous examples, and (near) duplicates.
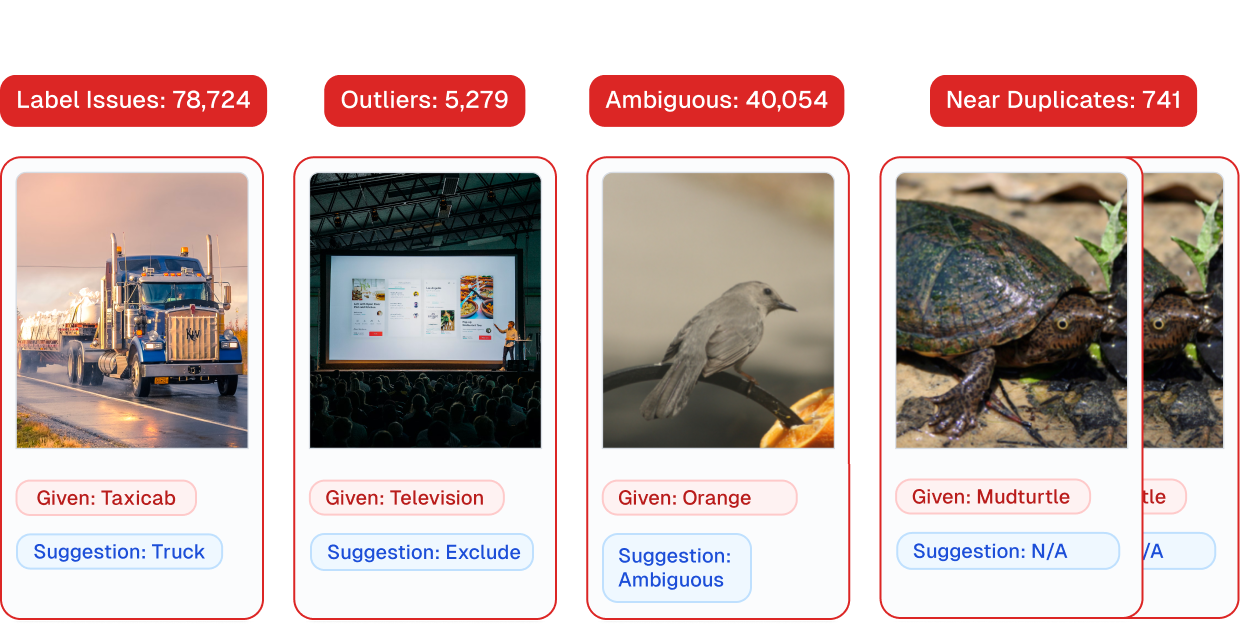
HOW IT WORKS
Auto-detect Issues
Automatically detect potential issues in image datasets like images that are: under/over-exposed, blurry, near duplicates, or low-information. Automatically detect outliers (anomalies) which may have an outsized impact on data-driven conclusions and should be handled with care.
Facilitate Agricultural Tasks
Cleanlab Studio facilitates data-centric AI workflows in both agricultural applications (i.e. disease inspection, yield estimation, animal monitoring, tasks involving grading and sorting) and Industrial quality control applications (i.e. ingredient inspection, process quality monitoring, assembly inspection, defect detection).
Built with AI
Use our ActiveLab system (active learning with relabeling) to efficiently collect new labels for training accurate models.
Quality Analysis
Know which subset of the data is high-quality with confidence, and evaluate the quality of different data sources.
Assessing Multiple Data Annotators
Effectively analyze data labeled by multiple annotators, and estimate which examples require additional review and which annotators are best/worst overall.
Resources and Tutorials
Videos on using Cleanlab Studio to find and fix incorrect labels for: text data, tabular data, and image data
CASE STUDY
Gojek is an Indonesian on-demand multi-service platform and digital payment technology group.
Gojek used Cleanlab to remove low-quality labels on an image dataset. The model trained on the dataset without low-quality data gained 4 percentage points of accuracy compared to the baseline model (trained on all data).
Read more in Travis Tang’s Toward AI article: Cleanlab: Correct your data labels automatically and quickly.
+14%
error improvement for ResNet computer vision model (without any change in modeling code)
“That’s why Cleanlab is valuable. Trust me. I spent a painful week meticulously checking labels trying to stay awake. You do not want to do that.”
Data Scientist at Gojek