How Cleanlab can help
Find tens of thousands of data errors.
Large-scale datasets used in enterprise data analytics and machine learning are often full of errors, leading to lower reliability, lost productivity, and increased costs. With Cleanlab, you can automatically find and fix issues in data at scale, effortlessly curating high-quality datasets.
ImageNet is the most famous computer vision (image recognition) dataset with millions of images. Cleanlab Studio automatically found thousands of data errors in their dataset, like label issues, outliers, ambiguous examples, and (near) duplicates.
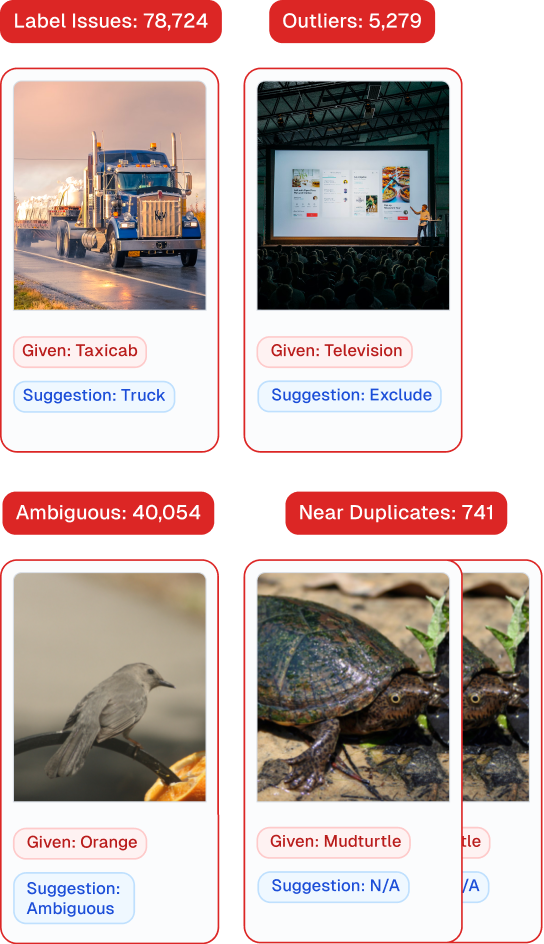
HOW IT WORKS
Auto-detect Issues
Automatically discover outliers (anomalies) lurking in any dataset. Detect low-quality examples in any image dataset.
Audit Data and Summarize Patterns
Audit data stored in many file formats: Excel, CSV, JSON, etc. including data with many raw text fields or images. Summarize overall patterns in data errors to better understand where they stem from and how they might affect conclusions.
Assessing Multiple Data Annotators
Reconcile conflicting decisions made by multiple data entry workers and discover which workers are best/worst overall. Learn more.
Train and Produce Reliable Models
Use Cleanlab AutoML to train and deploy state-of-the-art ML models in one click. Robustly train models on cleaned data to predict any information recorded in your dataset, no Machine Learning expertise required! This can help with missing value imputation and other tasks involving incomplete information.
Resources and Tutorials
Videos on using Cleanlab Studio to find and fix incorrect labels for: text annotation or metadata, image annotation or metadata, and data tables.
CASE STUDY
The Stakeholder Company’s mission is to build tech to connect our divided world by connecting the dots between what’s happening and who’s making it happen across the world’s most important issues.
Cleanlab was used to quickly validate one of The Stakeholder Company’s classifier models’ predictions for a dataset. This is typically a very time-consuming task, as they would normally have to check thousands of examples by hand, but the process was expedited by leveraging Cleanlab.
8x
reduction in time spent
“Since Cleanlab helped us identify the data points that were most likely to have label errors, we only had to inspect an eighth of our dataset to see that our model was problematic.”
Data Analyst at The Stakeholder Company