The volume of content generated today makes manual content moderation impossible. Teams using automated content moderation to provide safe user experiences must invest significantly in datasets and ML models. Without needing you to write any code, Cleanlab can automatically curate your content moderation datasets in order to improve your own ML models. Cleanlab also offers an AI system that can auto-detect more types of safety/quality issues than your existing content moderation models.
For instance, Cleanlab’s AI will flag any images that are: NSFW, blurry, low-information, over/under-exposed, odd size/shape, or otherwise unaesthetic — as well as any text that is: toxic/harmful, expressing bias/prejudice, PII, indecipherable, poorly written (slang/spelling/grammar), or in a foreign language. All of this can be accomplished within minutes in Cleanlab, enabling expansive content moderation that not only ensures safety and privacy, but also the quality of your content.
Read more: Automatically Detect Problematic Content in any Text Dataset and How to Filter Unsafe and Low-Quality Images From any Dataset
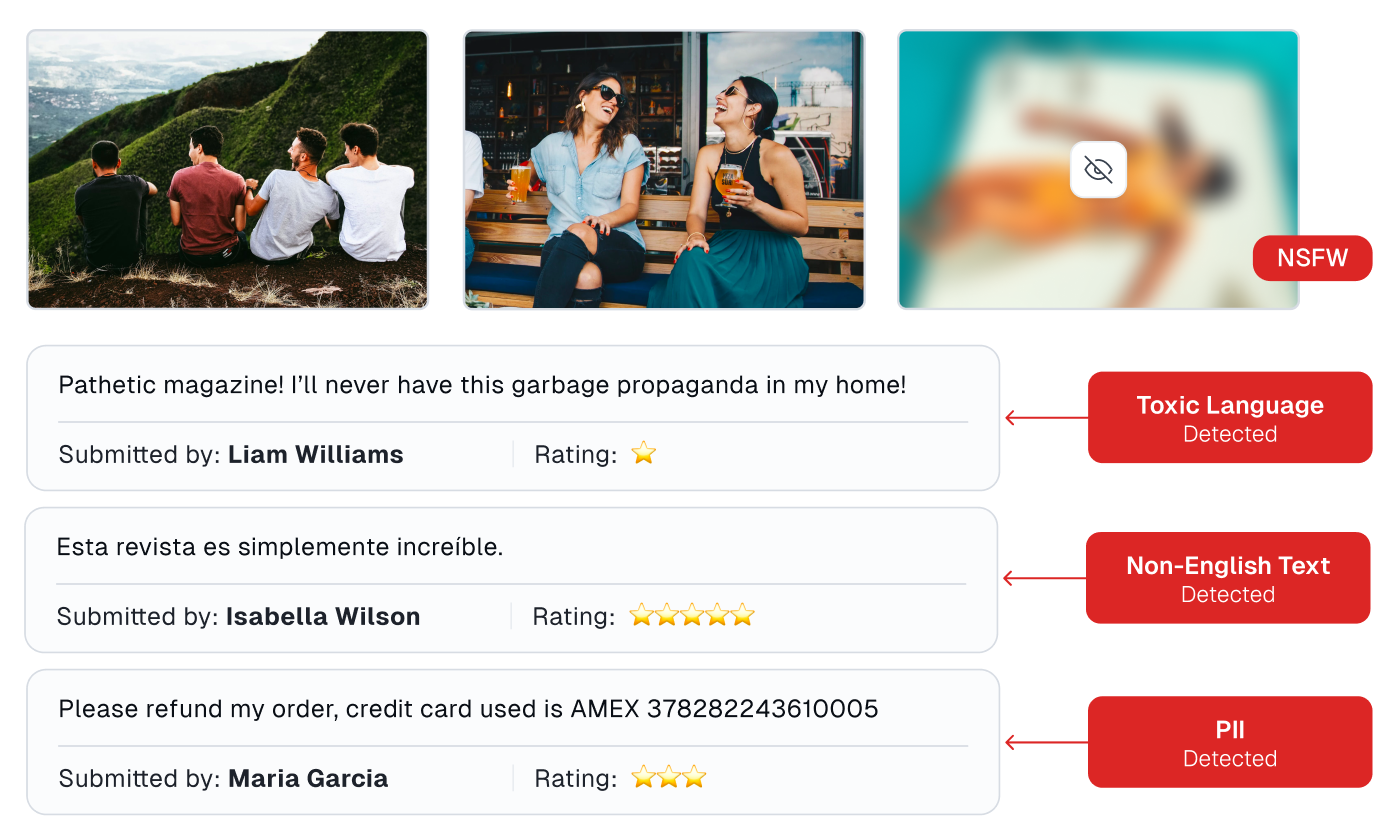
HOW IT WORKS
Catch Unsafe and Low-Quality Images in any Dataset
Read how Cleanlab Studio can be used as an automated solution to ensure high-quality image data, for both content moderation and boosting engagement. Curate any product/content catalog or photo gallery by automatically detecting images that are outliers, near duplicates, Not-Safe-For-Work, or low-quality (over/under-exposed, blurry, oddly-sized/distorted, low-information, or otherwise unaesthetic).
Catch Problematic Text in any Dataset
Read how Cleanlab Studio can be used for automated content moderation and curation of product descriptions and reviews. Cleanlab’s AI flags any text that is: poorly-written, non-English, indecipherable, or contains toxic language or Personally Identifiable Information.
Train and Produce Reliable Models
Train and deploy state-of-the-art content moderation/categorization models (with well-calibrated uncertainty estimates) in one click. Cleanlab Studio automatically applies the most suitable Foundation/LLM models and AutoML systems for your content.
Minimize Human Review
Confidently make model-assisted moderation decisions in real-time, deciding when to flag content for human review, and when to request a second/third review (for hard examples).
Assess Multiple Content Annotators
Determine which of your moderators is performing best/worst overall Read about analyzing politeness labels provided by multiple data annotators.
Resources and Tutorials
Videos on using Cleanlab Studio to find and fix incorrect labels for: text annotation or metadata, image annotation or metadata, and data tables.
CASE STUDY
Hometree Data (formely VAST-OSINT) is on a quest to tame the web into safe, secure and on-demand data streams to help customers isolate and remediate misinformation, detect influence operations, and keep your companies and customers safer.
Hometree Data used Cleanlab to identify incorrect labels to improve toxic language detection models.
“I took the sentence-labeled training data and threw it at Cleanlab to see how well confident learning could identify the incorrect labels. These results look amazing to me.”
CTO of Hometree Data
ShareChat is India’s largest native social media app with over 300 million users. The company employs large teams of content moderators to categorize user video content in many ways.
ShareChat uses Cleanlab to improve content classification. For this dataset, Cleanlab Studio’s AutoML automatically produced a more accurate visual concept classification model (56.7% accuracy) than ShareChat’s in-house 3D ResNet model (52.6% accuracy). Automated data curation of Sharechat’s dataset with Cleanlab immediately reduced ML error-rates by 5% (no change in the ML model).
+3%
error rate automatically identified by Cleanlab