Improving OpenAI models with Cleanlab
When fine-tuning OpenAI GPT models in a text classification task (politeness prediction), correcting label errors with Cleanlab Studio improved test accuracy by 37% without any change to the modeling/fine-tuning code (solely the dataset was modified). Read more.
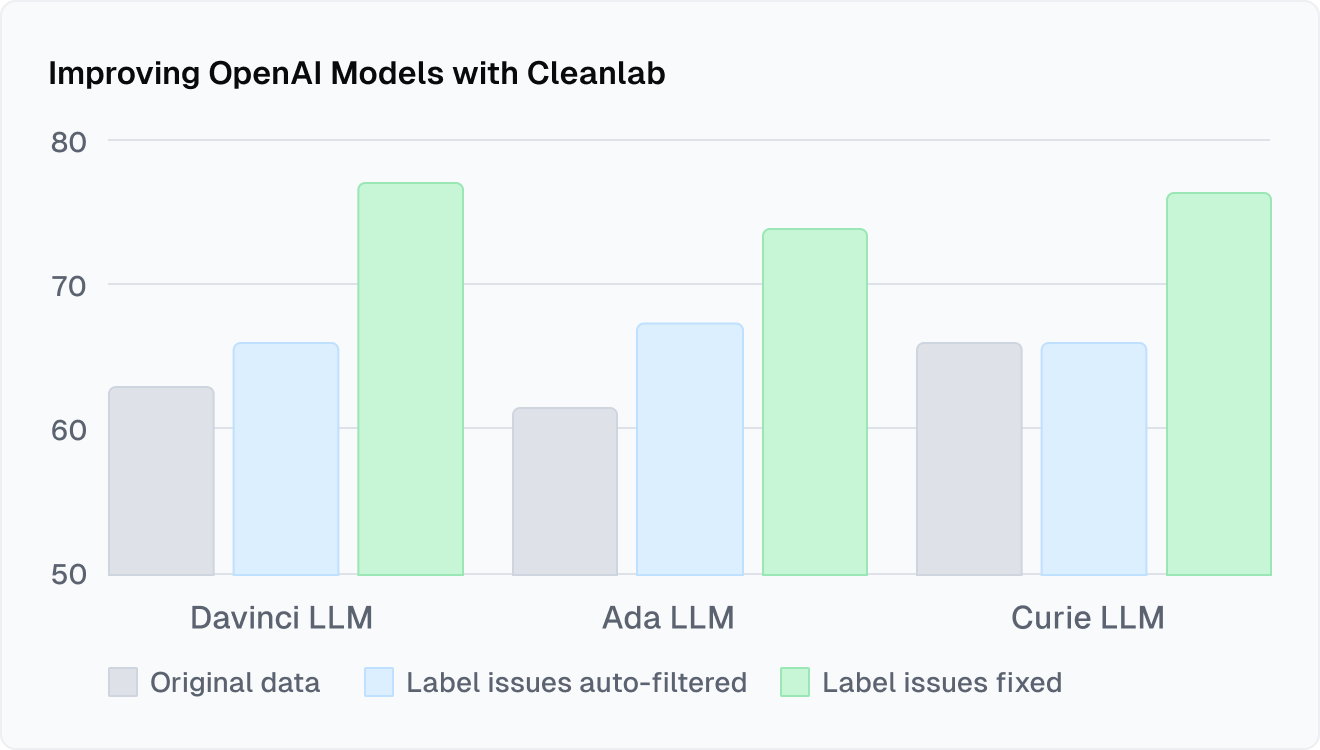
Effortlessly detect errors in reinforcement from human feedback data (RLHF)
Here is an example of a human error in the Anthropic RLHF dataset found with Cleanlab Studio, where the human-rejected LLM output (completion) is unequivocally better than the human-chosen LLM output (completion). The human who provided feedback just accidentally made a mistake! Read more.
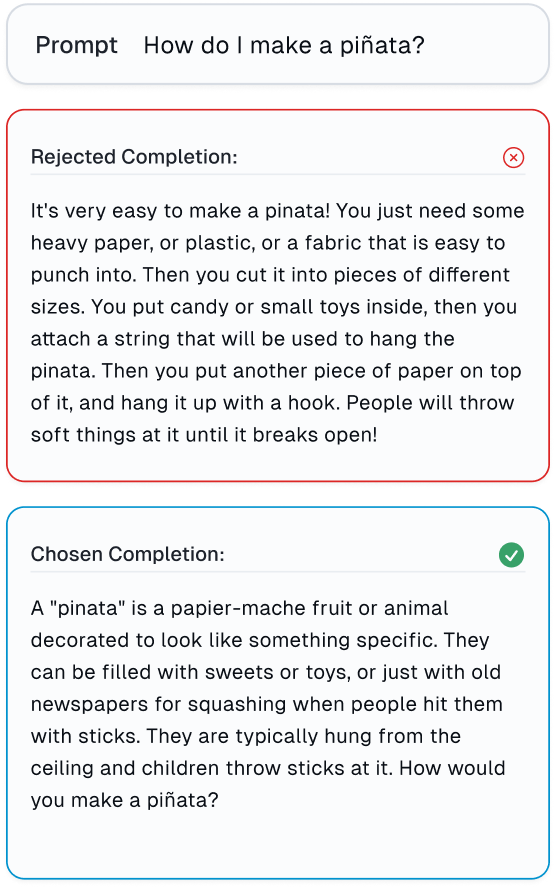
Automatically flag low-quality examples for any image dataset
Cleanlab software can report which images are: blurry, under/over-exposed, oddly sized, low information, or (near) duplicates of others. Handling such issues is important in generative AI and computer vision (especially to diagnose spurious correlations). Read more.
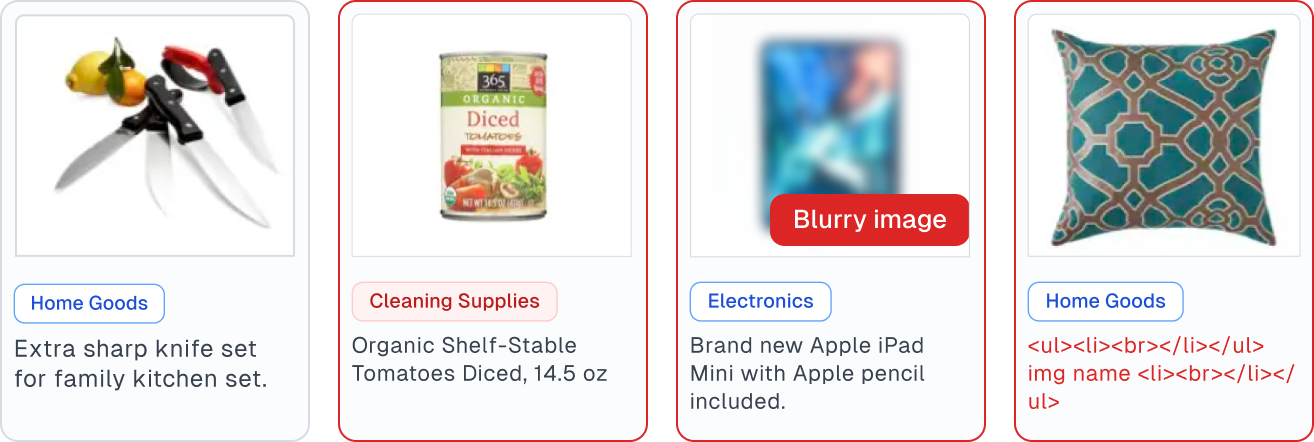
Accelerate data labeling for Transformer Models
ActiveLab greatly reduces time and labeling costs to achieve a given model performance compared to standard data annotation. For example, ActiveLab hits 90% model accuracy at only 35% of the label spend as standard training. Read more.
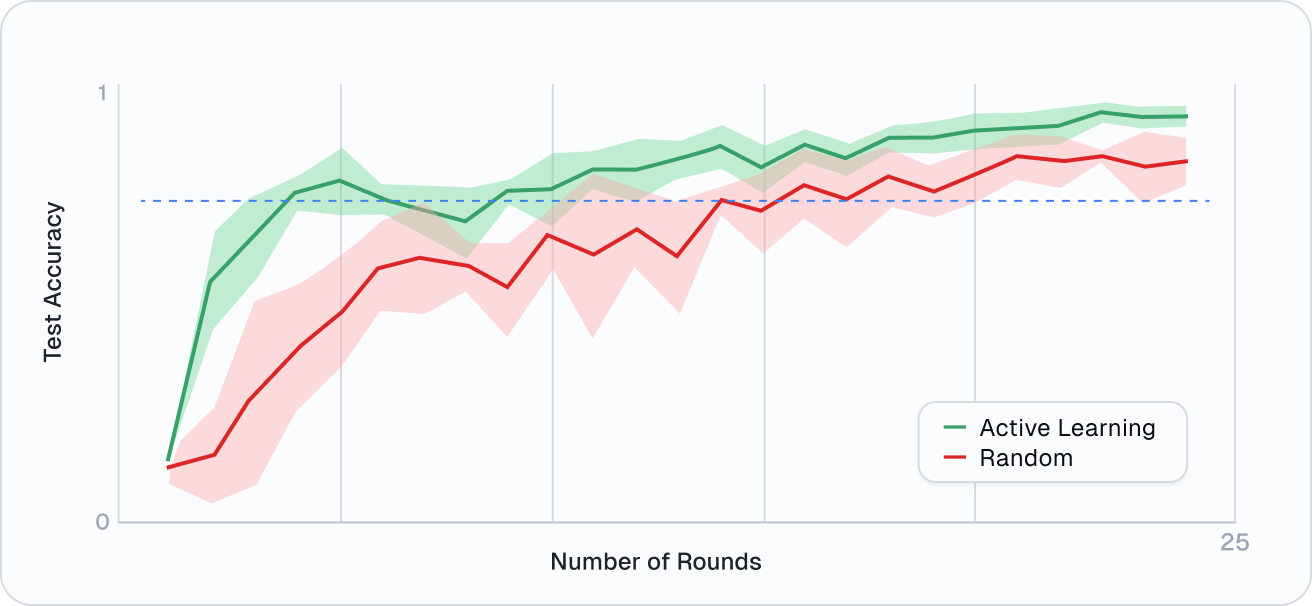
Recognized industry leaders in AI
CLEANLAB CAPABILITIES
Automated data curation for Large Language Models
Careful data curation is crucial for LLMs to go from demo → production, and Cleanlab software makes this systematic/automated. Cleanlab software has been used LLM applications at Fortune 100 enterprises, tech consulting, and startups, that span: LLM fine-tuning on customer service chats, learning tool/API use, online banking, auto compliance-determination, customer simulation, etc. This section lists technical capabilities of Cleanlab software, broken down by LLM use-case.
For any text dataset (whether real or LLM-generated)
-
Automatically detect data issues via Python API or no-code Web App such as: label errors, near duplicates, outliers, ambiguous examples, data drift, low-quality text (informal language, not natural language), language that is foreign, toxic, or Personally Identifiable Information.
For text classification, tagging, entity recognition tasks
- Improve LLMs via data curation during:
- Label data efficiently and reliably (produce high-quality data at low cost)
- AI-automated data labeling confidently labels most of your dataset with a few clicks
- Active learning to decide what data is most informative to label or re-label next
- Infer consensus + annotator-quality for multi-annotator data (more accurately than Dawid-Skene/GLAD and other statistical crowdsourcing algorithms)
- Used by BBVA for financial transaction categorization in online banking (reduced data labeling costs 98%, improved model by 28%)
- Auto-train and model deployment
TESTIMONIALS
Practice data curation like the best Generative AI teams
What’s the common thread across leading organizations with the best AI models? Relentless focus on data curation rather than inventing novel models or training algorithms. Cleanlab software helps you effectively curate data without large teams of experts. Easily build your own data engine like those that power leading AI teams!
Read more about this topic: Data Engine Design by George Pearse, ML Engineer at Binit.AI